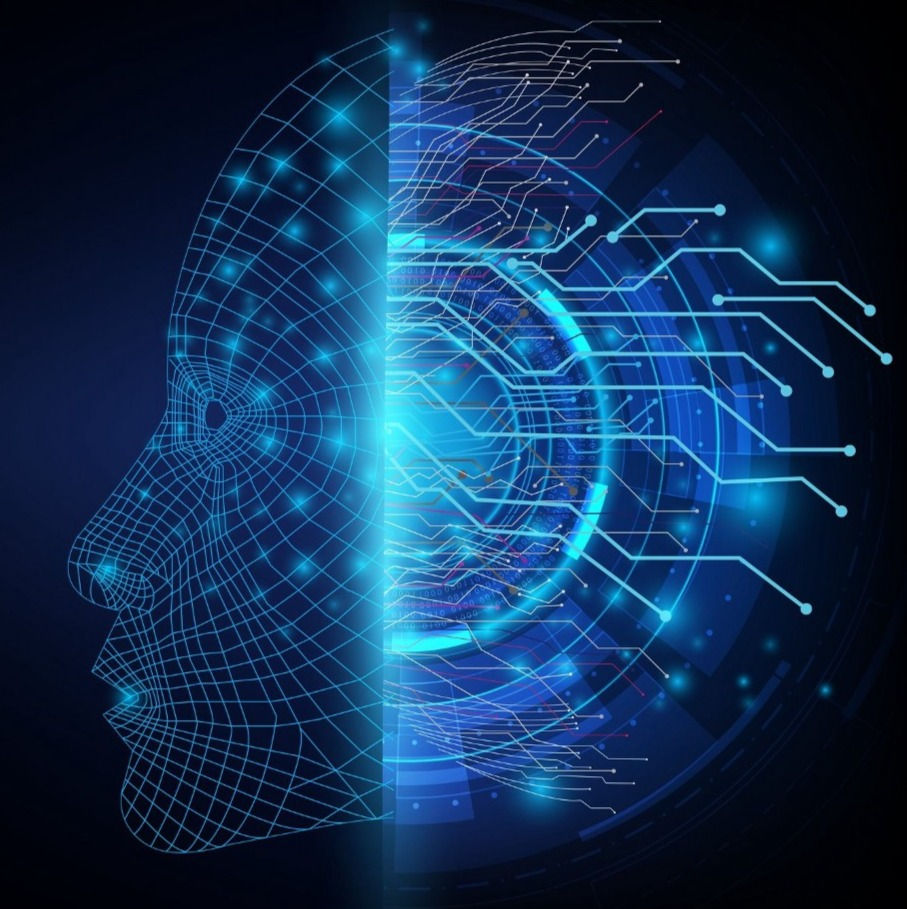
What exactly is Federated Learning, and why are innovators and early adopters so excited about it?
Chances are that if you like to stay on the cutting edge in the artificial intelligence and machine learning areas that you've at least heard about federated learning. But why is it such a big deal, and who should be investing in it now?
In federated learning, a machine learning model is deployed on a device. It uses the data it gathers, trains on it, and then only sends the valuable intelligence to be collected together with the intelligence of other devices. Remember, no data is sent, only the intelligence.
With all of the intelligence that is shared and collected, a smarter model is created, and then sent back to each device, allowing it to become much more intelligent. This process is then repeated over and over.
“no data leaves the device, only the valuable intelligence..”
Now, let's contrast this with the current approach of handling data. Generally, data is collected in some form, and sent to one central location. Here all the data is used to train models and to ultimately learn something useful (intelligence). There are many problems and challenges with this.
First, data accessibility is difficult, cumbersome, and sometimes not possible. Sending all data could be a violation of privacy regulations. We've all heard about organizations databases being hacked and then personal information being leaked out, available for anyone to access. Not only is this potentially extremely costly for the personal lives of those whose information was hacked, but also devastating to the business that was charged with protecting it. As more and more regulations are put in place to prevent this from happening (and rightly so), businesses are having an increasingly difficult time gathering value from this data.
The second challenge with this current approach is the sheer amount of data that needs to be sent to the central location. Imagine operating a gold mine, and hauling all of the dirt hundreds of miles, only then processing it to extract the gold. This wouldn't be necessary if you were able to process on site.
The costs to do this are quite high. Of course, the amount of data sent can vary greatly from business to business. To provide some context, according to datafloq.com, the company Electronic Arts processes roughly 50 terabytes of data every day.
Two more trends will continue to drive this much higher in the coming years. It's been estimated that there are roughly 26 billion IoT devices creating data. According to many sources, this number will more than triple to 76 billion devices by 2025. Also, let's consider what the advent of 5G will contribute. 5G was designed to process roughly one hundred times more data than current 4G networks.
Add to this the significant additional cost of storing this data. Beyond just the financial costs, this method is also a waste of valuable energy. In a time where businesses have taken a closer look at how their own impact on the environment and their carbon footprint, it makes sense to look for ways to be more efficient.
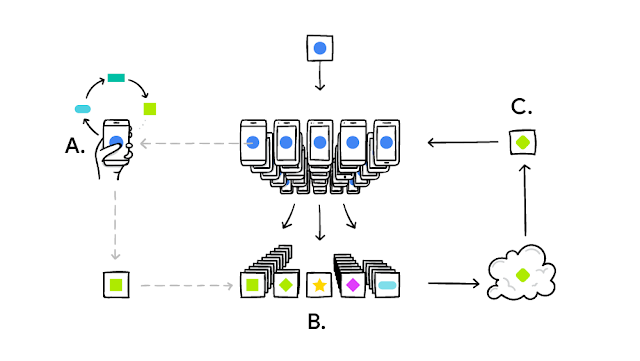
Credit: ai.googleblog.com
The example above is a great illustration of how federated learning works. Imagine a phone user. We can use a model to train on personal behavior (A). Only the intelligence from this model ( no data ) is then sent to be aggregated (collected) with the intelligence from other personal behavior models (B). Finally the updated intelligence from all models is then sent back to the original device, as a much smarter model is realized.
The process is then repeated over and over. Again, the main point here is that no data leaves the device, only the valuable intelligence.
Want to learn more?
If you're ready to see what federated learning can do for you and your business, you can get started with a Free Trial of our STADLE Platform by clicking here.
If you want to discuss ways that FL can help you or your business, please reach out to us, we'd love to talk. Click here to contact us.
Comentários